Author: perezparedes
Is Getting a Dog The First Step To Parenthood?
A Large Title
Lorem ipsum dolor sit amet, consectetuer adipiscing elit. Aenean commodo ligula eget dolor. Aenean massa. Cum sociis natoque penatibus et magnis dis parturient montes, nascetur ridiculus mus. Donec quam felis, ultricies nec, pellentesque eu, pretium quis, sem. Nulla consequat massa quis enim. Donec pede justo, fringilla vel, aliquet nec, vulputate eget, arcu. In enim justo, rhoncus ut, imperdiet a, venenatis vitae, justo. Nullam dictum felis eu pede mollis pretium. Integer tincidunt. Cras dapibus. Vivamus elementum semper nisi.
Procrastination in just an exceptional sense of understanding about when things should actually happen.
Tim Lemons
The Smaller Title
Aenean vulputate eleifend tellus. Aenean leo ligula, porttitor eu, consequat vitae, eleifend ac, enim. Aliquam lorem ante, dapibus in, viverra quis, feugiat a, tellus. Phasellus viverra nulla ut metus varius laoreet. Quisque rutrum. Aenean imperdiet. Etiam ultricies nisi vel augue. Curabitur ullamcorper ultricies nisi. Nam eget dui. Etiam rhoncus. Maecenas tempus, tellus eget condimentum rhoncus, sem quam semper libero, sit amet adipiscing sem neque sed ipsum. Nam quam nunc, blandit vel, luctus pulvinar, hendrerit id, lorem. Maecenas nec odio et ante tincidunt tempus.
- Bullet points make a great dot
- Great way to make a point
- A well-sorted fact
- A very informative black spot
- Start with One
- Then proceed to Dos
- Hello from Japan – San
- This is Quatre
Aenean vulputate eleifend tellus. Aenean leo ligula, porttitor eu, consequat vitae, eleifend ac, enim. Aliquam lorem ante, dapibus in, viverra quis, feugiat a, tellus. Phasellus viverra nulla ut metus varius laoreet. Quisque rutrum. Aenean imperdiet. Etiam ultricies nisi vel augue. Curabitur ullamcorper ultricies nisi. Nam eget dui. Etiam rhoncus. Maecenas tempus, tellus eget condimentum rhoncus, sem quam semper libero, sit amet adipiscing sem neque sed ipsum. Nam quam nunc, blandit vel, luctus pulvinar, hendrerit id, lorem. Maecenas nec odio et ante tincidunt tempus.
If life gives you lemons.. make an elaborate corporate lemon juice pyramid scheme.
Lemmony Meadow
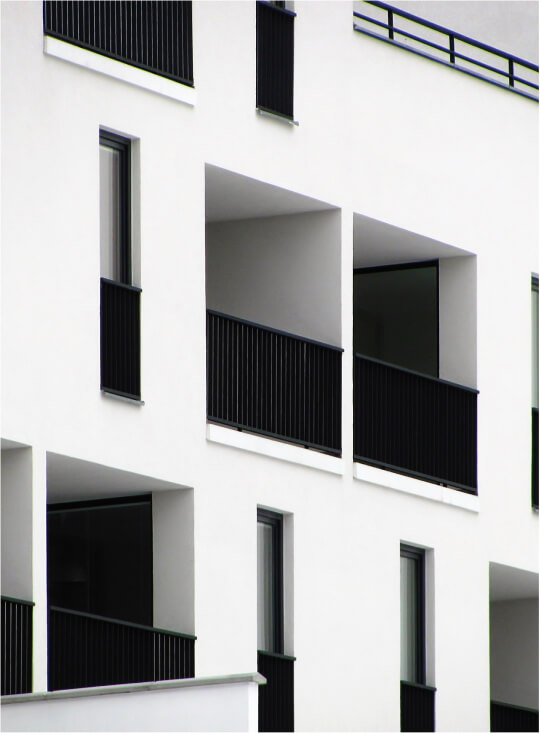
The image on the left
Lorem ipsum dolor sit amet, consectetur adipiscing elit. Aenean a orci bibendum, egestas quam et, mollis massa. Nam id diam et lacus facilisis viverra in quis metus. Donec nibh mi, porttitor scelerisque molestie ac, pharetra vitae arcu. Morbi sollicitudin, arcu non dignissim luctus, risus massa rhoncus dolor, ut accumsan diam nulla sit amet neque. Etiam quam nibh, porta
The image on the right
Lorem ipsum dolor sit amet, consectetur adipiscing elit. Aenean a orci bibendum, egestas quam et, mollis massa. Nam id diam et lacus facilisis viverra in quis metus. Donec nibh mi, porttitor scelerisque molestie ac, pharetra vitae arcu. Morbi sollicitudin, arcu non dignissim luctus, risus massa rhoncus dolor, ut accumsan diam nulla sit amet neque. Etiam quam nibh, porta ac diam maximus, porta sagittis ex. Cras ac lectus sit amet orci sodales molestie. Morbi interdum lectus in porta pharetra.
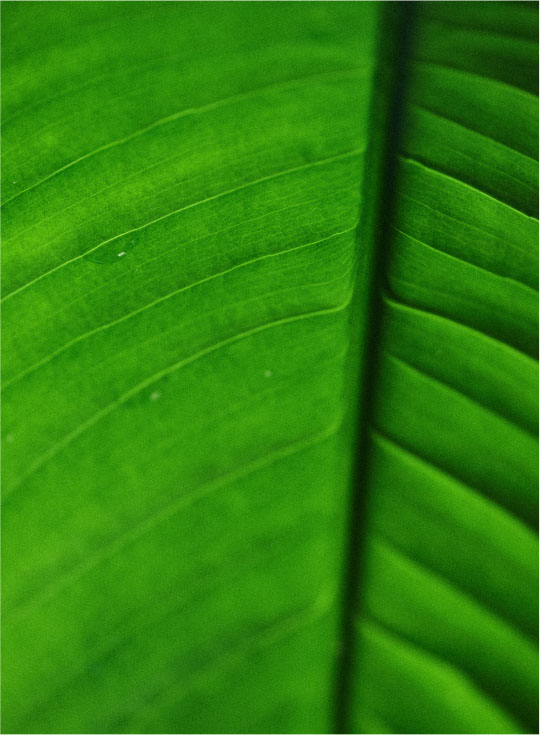
What You Should Know When Making a Showcase For Your Products
A Large Title
Lorem ipsum dolor sit amet, consectetuer adipiscing elit. Aenean commodo ligula eget dolor. Aenean massa. Cum sociis natoque penatibus et magnis dis parturient montes, nascetur ridiculus mus. Donec quam felis, ultricies nec, pellentesque eu, pretium quis, sem. Nulla consequat massa quis enim. Donec pede justo, fringilla vel, aliquet nec, vulputate eget, arcu. In enim justo, rhoncus ut, imperdiet a, venenatis vitae, justo. Nullam dictum felis eu pede mollis pretium. Integer tincidunt. Cras dapibus. Vivamus elementum semper nisi.
Procrastination in just an exceptional sense of understanding about when things should actually happen.
Tim Lemons
The Smaller Title
Aenean vulputate eleifend tellus. Aenean leo ligula, porttitor eu, consequat vitae, eleifend ac, enim. Aliquam lorem ante, dapibus in, viverra quis, feugiat a, tellus. Phasellus viverra nulla ut metus varius laoreet. Quisque rutrum. Aenean imperdiet. Etiam ultricies nisi vel augue. Curabitur ullamcorper ultricies nisi. Nam eget dui. Etiam rhoncus. Maecenas tempus, tellus eget condimentum rhoncus, sem quam semper libero, sit amet adipiscing sem neque sed ipsum. Nam quam nunc, blandit vel, luctus pulvinar, hendrerit id, lorem. Maecenas nec odio et ante tincidunt tempus.
- Bullet points make a great dot
- Great way to make a point
- A well-sorted fact
- A very informative black spot
- Start with One
- Then proceed to Dos
- Hello from Japan – San
- This is Quatre
Aenean vulputate eleifend tellus. Aenean leo ligula, porttitor eu, consequat vitae, eleifend ac, enim. Aliquam lorem ante, dapibus in, viverra quis, feugiat a, tellus. Phasellus viverra nulla ut metus varius laoreet. Quisque rutrum. Aenean imperdiet. Etiam ultricies nisi vel augue. Curabitur ullamcorper ultricies nisi. Nam eget dui. Etiam rhoncus. Maecenas tempus, tellus eget condimentum rhoncus, sem quam semper libero, sit amet adipiscing sem neque sed ipsum. Nam quam nunc, blandit vel, luctus pulvinar, hendrerit id, lorem. Maecenas nec odio et ante tincidunt tempus.
If life gives you lemons.. make an elaborate corporate lemon juice pyramid scheme.
Lemmony Meadow
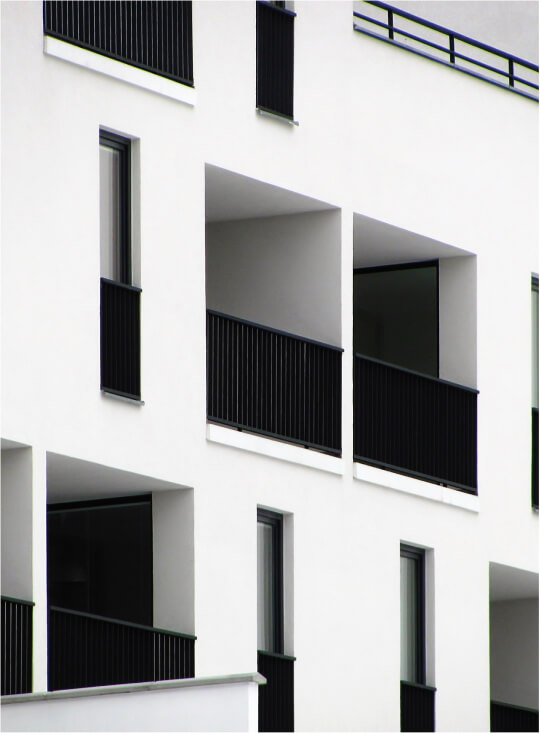
The image on the left
Lorem ipsum dolor sit amet, consectetur adipiscing elit. Aenean a orci bibendum, egestas quam et, mollis massa. Nam id diam et lacus facilisis viverra in quis metus. Donec nibh mi, porttitor scelerisque molestie ac, pharetra vitae arcu. Morbi sollicitudin, arcu non dignissim luctus, risus massa rhoncus dolor, ut accumsan diam nulla sit amet neque. Etiam quam nibh, porta
The image on the right
Lorem ipsum dolor sit amet, consectetur adipiscing elit. Aenean a orci bibendum, egestas quam et, mollis massa. Nam id diam et lacus facilisis viverra in quis metus. Donec nibh mi, porttitor scelerisque molestie ac, pharetra vitae arcu. Morbi sollicitudin, arcu non dignissim luctus, risus massa rhoncus dolor, ut accumsan diam nulla sit amet neque. Etiam quam nibh, porta ac diam maximus, porta sagittis ex. Cras ac lectus sit amet orci sodales molestie. Morbi interdum lectus in porta pharetra.
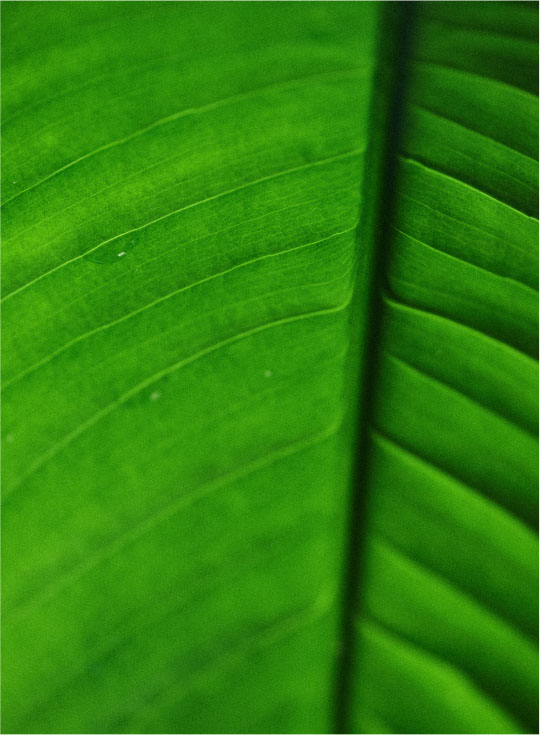
Talking to Inanimate Objects Made Me a Better Designer
A Large Title
Lorem ipsum dolor sit amet, consectetuer adipiscing elit. Aenean commodo ligula eget dolor. Aenean massa. Cum sociis natoque penatibus et magnis dis parturient montes, nascetur ridiculus mus. Donec quam felis, ultricies nec, pellentesque eu, pretium quis, sem. Nulla consequat massa quis enim. Donec pede justo, fringilla vel, aliquet nec, vulputate eget, arcu. In enim justo, rhoncus ut, imperdiet a, venenatis vitae, justo. Nullam dictum felis eu pede mollis pretium. Integer tincidunt. Cras dapibus. Vivamus elementum semper nisi.
Procrastination in just an exceptional sense of understanding about when things should actually happen.
Tim Lemons
The Smaller Title
Aenean vulputate eleifend tellus. Aenean leo ligula, porttitor eu, consequat vitae, eleifend ac, enim. Aliquam lorem ante, dapibus in, viverra quis, feugiat a, tellus. Phasellus viverra nulla ut metus varius laoreet. Quisque rutrum. Aenean imperdiet. Etiam ultricies nisi vel augue. Curabitur ullamcorper ultricies nisi. Nam eget dui. Etiam rhoncus. Maecenas tempus, tellus eget condimentum rhoncus, sem quam semper libero, sit amet adipiscing sem neque sed ipsum. Nam quam nunc, blandit vel, luctus pulvinar, hendrerit id, lorem. Maecenas nec odio et ante tincidunt tempus.
- Bullet points make a great dot
- Great way to make a point
- A well-sorted fact
- A very informative black spot
- Start with One
- Then proceed to Dos
- Hello from Japan – San
- This is Quatre
Aenean vulputate eleifend tellus. Aenean leo ligula, porttitor eu, consequat vitae, eleifend ac, enim. Aliquam lorem ante, dapibus in, viverra quis, feugiat a, tellus. Phasellus viverra nulla ut metus varius laoreet. Quisque rutrum. Aenean imperdiet. Etiam ultricies nisi vel augue. Curabitur ullamcorper ultricies nisi. Nam eget dui. Etiam rhoncus. Maecenas tempus, tellus eget condimentum rhoncus, sem quam semper libero, sit amet adipiscing sem neque sed ipsum. Nam quam nunc, blandit vel, luctus pulvinar, hendrerit id, lorem. Maecenas nec odio et ante tincidunt tempus.
If life gives you lemons.. make an elaborate corporate lemon juice pyramid scheme.
Lemmony Meadow
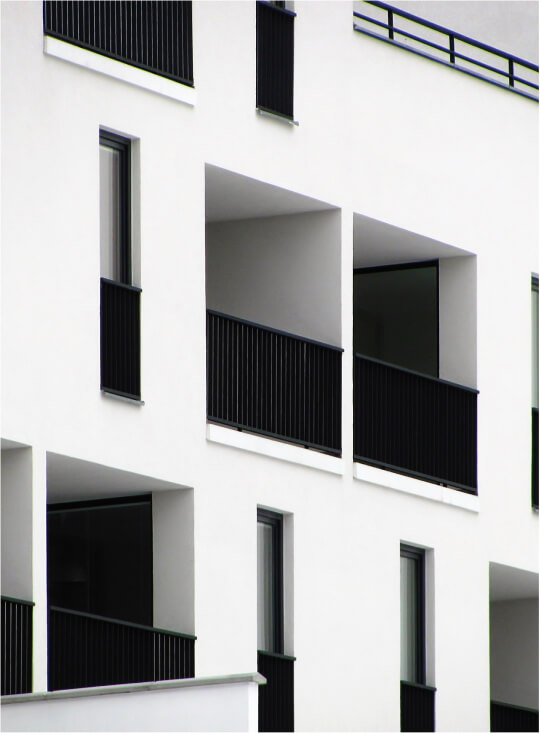
The image on the left
Lorem ipsum dolor sit amet, consectetur adipiscing elit. Aenean a orci bibendum, egestas quam et, mollis massa. Nam id diam et lacus facilisis viverra in quis metus. Donec nibh mi, porttitor scelerisque molestie ac, pharetra vitae arcu. Morbi sollicitudin, arcu non dignissim luctus, risus massa rhoncus dolor, ut accumsan diam nulla sit amet neque. Etiam quam nibh, porta
The image on the right
Lorem ipsum dolor sit amet, consectetur adipiscing elit. Aenean a orci bibendum, egestas quam et, mollis massa. Nam id diam et lacus facilisis viverra in quis metus. Donec nibh mi, porttitor scelerisque molestie ac, pharetra vitae arcu. Morbi sollicitudin, arcu non dignissim luctus, risus massa rhoncus dolor, ut accumsan diam nulla sit amet neque. Etiam quam nibh, porta ac diam maximus, porta sagittis ex. Cras ac lectus sit amet orci sodales molestie. Morbi interdum lectus in porta pharetra.
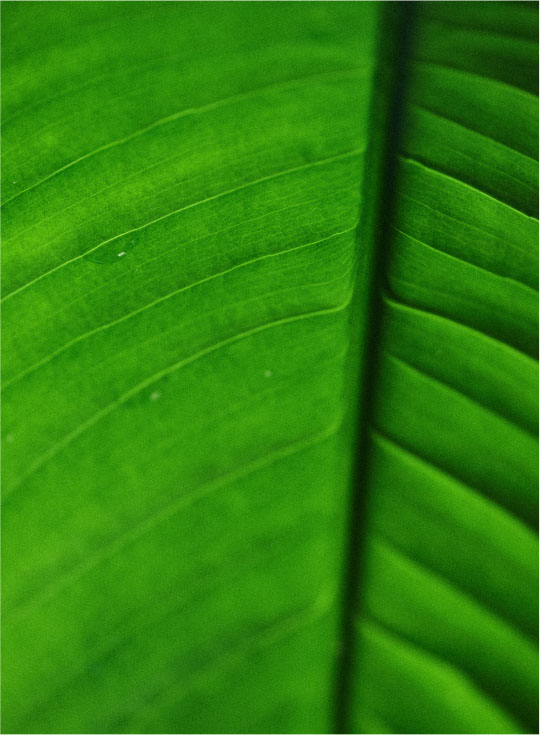
How to Stay Productive When Working From Home
A Large Title
Lorem ipsum dolor sit amet, consectetuer adipiscing elit. Aenean commodo ligula eget dolor. Aenean massa. Cum sociis natoque penatibus et magnis dis parturient montes, nascetur ridiculus mus. Donec quam felis, ultricies nec, pellentesque eu, pretium quis, sem. Nulla consequat massa quis enim. Donec pede justo, fringilla vel, aliquet nec, vulputate eget, arcu. In enim justo, rhoncus ut, imperdiet a, venenatis vitae, justo. Nullam dictum felis eu pede mollis pretium. Integer tincidunt. Cras dapibus. Vivamus elementum semper nisi.
Procrastination in just an exceptional sense of understanding about when things should actually happen.
Tim Lemons
The Smaller Title
Aenean vulputate eleifend tellus. Aenean leo ligula, porttitor eu, consequat vitae, eleifend ac, enim. Aliquam lorem ante, dapibus in, viverra quis, feugiat a, tellus. Phasellus viverra nulla ut metus varius laoreet. Quisque rutrum. Aenean imperdiet. Etiam ultricies nisi vel augue. Curabitur ullamcorper ultricies nisi. Nam eget dui. Etiam rhoncus. Maecenas tempus, tellus eget condimentum rhoncus, sem quam semper libero, sit amet adipiscing sem neque sed ipsum. Nam quam nunc, blandit vel, luctus pulvinar, hendrerit id, lorem. Maecenas nec odio et ante tincidunt tempus.
- Bullet points make a great dot
- Great way to make a point
- A well-sorted fact
- A very informative black spot
- Start with One
- Then proceed to Dos
- Hello from Japan – San
- This is Quatre
Aenean vulputate eleifend tellus. Aenean leo ligula, porttitor eu, consequat vitae, eleifend ac, enim. Aliquam lorem ante, dapibus in, viverra quis, feugiat a, tellus. Phasellus viverra nulla ut metus varius laoreet. Quisque rutrum. Aenean imperdiet. Etiam ultricies nisi vel augue. Curabitur ullamcorper ultricies nisi. Nam eget dui. Etiam rhoncus. Maecenas tempus, tellus eget condimentum rhoncus, sem quam semper libero, sit amet adipiscing sem neque sed ipsum. Nam quam nunc, blandit vel, luctus pulvinar, hendrerit id, lorem. Maecenas nec odio et ante tincidunt tempus.
If life gives you lemons.. make an elaborate corporate lemon juice pyramid scheme.
Lemmony Meadow
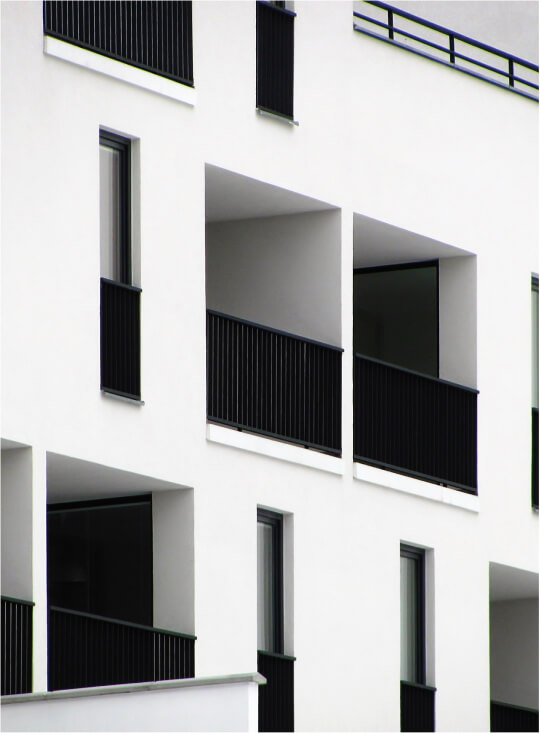
The image on the left
Lorem ipsum dolor sit amet, consectetur adipiscing elit. Aenean a orci bibendum, egestas quam et, mollis massa. Nam id diam et lacus facilisis viverra in quis metus. Donec nibh mi, porttitor scelerisque molestie ac, pharetra vitae arcu. Morbi sollicitudin, arcu non dignissim luctus, risus massa rhoncus dolor, ut accumsan diam nulla sit amet neque. Etiam quam nibh, porta
The image on the right
Lorem ipsum dolor sit amet, consectetur adipiscing elit. Aenean a orci bibendum, egestas quam et, mollis massa. Nam id diam et lacus facilisis viverra in quis metus. Donec nibh mi, porttitor scelerisque molestie ac, pharetra vitae arcu. Morbi sollicitudin, arcu non dignissim luctus, risus massa rhoncus dolor, ut accumsan diam nulla sit amet neque. Etiam quam nibh, porta ac diam maximus, porta sagittis ex. Cras ac lectus sit amet orci sodales molestie. Morbi interdum lectus in porta pharetra.
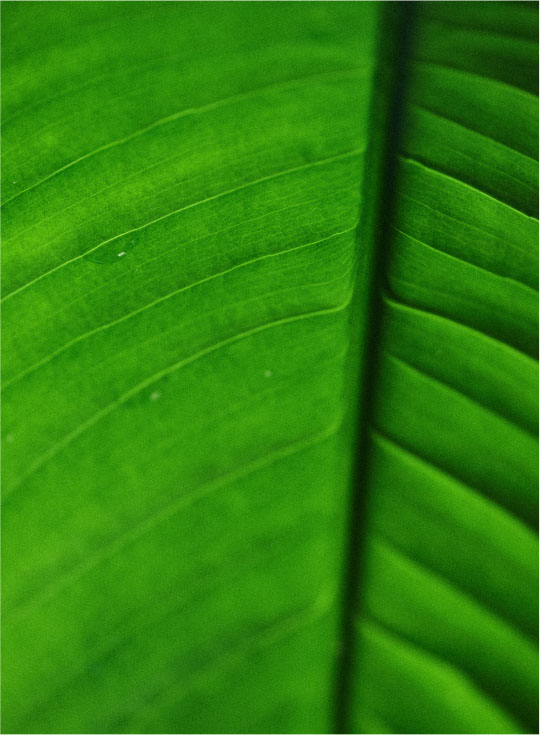
Is Design Becoming Too Minimalistic in 2023?
A Large Title
Lorem ipsum dolor sit amet, consectetuer adipiscing elit. Aenean commodo ligula eget dolor. Aenean massa. Cum sociis natoque penatibus et magnis dis parturient montes, nascetur ridiculus mus. Donec quam felis, ultricies nec, pellentesque eu, pretium quis, sem. Nulla consequat massa quis enim. Donec pede justo, fringilla vel, aliquet nec, vulputate eget, arcu. In enim justo, rhoncus ut, imperdiet a, venenatis vitae, justo. Nullam dictum felis eu pede mollis pretium. Integer tincidunt. Cras dapibus. Vivamus elementum semper nisi.
Procrastination in just an exceptional sense of understanding about when things should actually happen.
Tim Lemons
The Smaller Title
Aenean vulputate eleifend tellus. Aenean leo ligula, porttitor eu, consequat vitae, eleifend ac, enim. Aliquam lorem ante, dapibus in, viverra quis, feugiat a, tellus. Phasellus viverra nulla ut metus varius laoreet. Quisque rutrum. Aenean imperdiet. Etiam ultricies nisi vel augue. Curabitur ullamcorper ultricies nisi. Nam eget dui. Etiam rhoncus. Maecenas tempus, tellus eget condimentum rhoncus, sem quam semper libero, sit amet adipiscing sem neque sed ipsum. Nam quam nunc, blandit vel, luctus pulvinar, hendrerit id, lorem. Maecenas nec odio et ante tincidunt tempus.
- Bullet points make a great dot
- Great way to make a point
- A well-sorted fact
- A very informative black spot
- Start with One
- Then proceed to Dos
- Hello from Japan – San
- This is Quatre
Aenean vulputate eleifend tellus. Aenean leo ligula, porttitor eu, consequat vitae, eleifend ac, enim. Aliquam lorem ante, dapibus in, viverra quis, feugiat a, tellus. Phasellus viverra nulla ut metus varius laoreet. Quisque rutrum. Aenean imperdiet. Etiam ultricies nisi vel augue. Curabitur ullamcorper ultricies nisi. Nam eget dui. Etiam rhoncus. Maecenas tempus, tellus eget condimentum rhoncus, sem quam semper libero, sit amet adipiscing sem neque sed ipsum. Nam quam nunc, blandit vel, luctus pulvinar, hendrerit id, lorem. Maecenas nec odio et ante tincidunt tempus.
If life gives you lemons.. make an elaborate corporate lemon juice pyramid scheme.
Lemmony Meadow
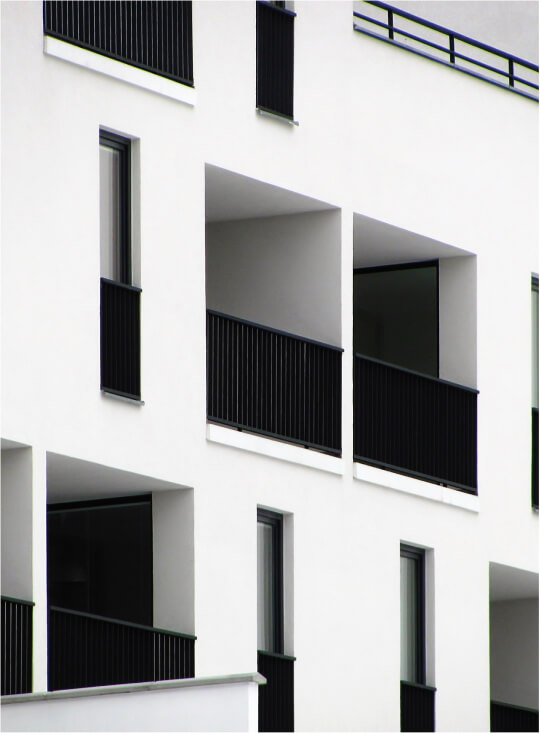
The image on the left
Lorem ipsum dolor sit amet, consectetur adipiscing elit. Aenean a orci bibendum, egestas quam et, mollis massa. Nam id diam et lacus facilisis viverra in quis metus. Donec nibh mi, porttitor scelerisque molestie ac, pharetra vitae arcu. Morbi sollicitudin, arcu non dignissim luctus, risus massa rhoncus dolor, ut accumsan diam nulla sit amet neque. Etiam quam nibh, porta
The image on the right
Lorem ipsum dolor sit amet, consectetur adipiscing elit. Aenean a orci bibendum, egestas quam et, mollis massa. Nam id diam et lacus facilisis viverra in quis metus. Donec nibh mi, porttitor scelerisque molestie ac, pharetra vitae arcu. Morbi sollicitudin, arcu non dignissim luctus, risus massa rhoncus dolor, ut accumsan diam nulla sit amet neque. Etiam quam nibh, porta ac diam maximus, porta sagittis ex. Cras ac lectus sit amet orci sodales molestie. Morbi interdum lectus in porta pharetra.
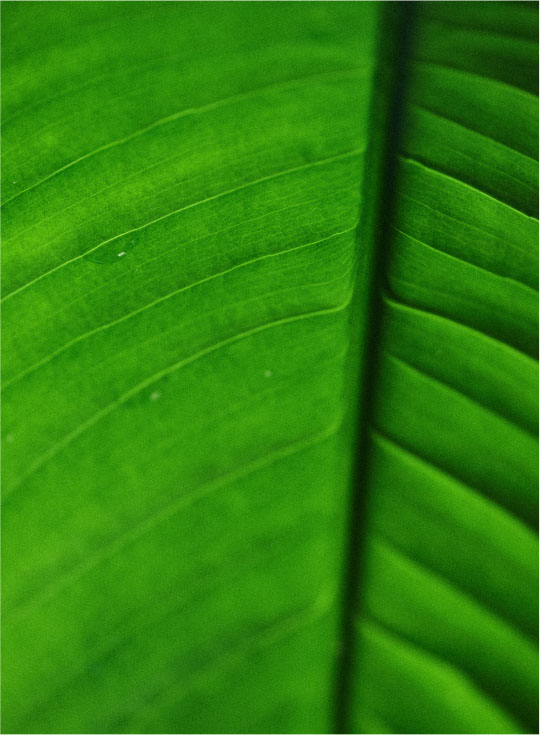